ChatGPT vs. Traditional NLP Models
- Sachin Pandey
- Sep 18, 2024
- 3 min read
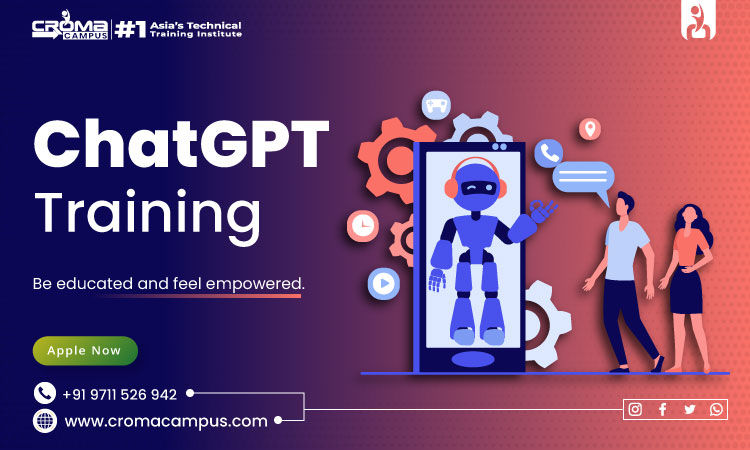
ChatGPT vs. Traditional NLP Models
Natural Language Processing (NLP) has made significant strides over the years, with advancements in models that understand and generate human language. ChatGPT, developed by OpenAI, represents a major leap forward in NLP, offering capabilities that traditional models struggle to match. This article explores how ChatGPT contrasts with traditional NLP models, focusing on its capabilities, advantages, and the impact of such advancements on the field. For those looking to delve deeper into these technologies, a ChatGPT Course and Prompt Engineer offer specialised training to harness these cutting-edge tools.
ChatGPT: A New Era in NLP
ChatGPT is based on the GPT (Generative Pre-trained Transformer) architecture, which leverages deep learning and large-scale datasets to generate human-like text. Unlike traditional NLP models, which often rely on rule-based or simpler machine learning techniques, ChatGPT utilizes the Transformer architecture to understand the context and generate coherent responses.
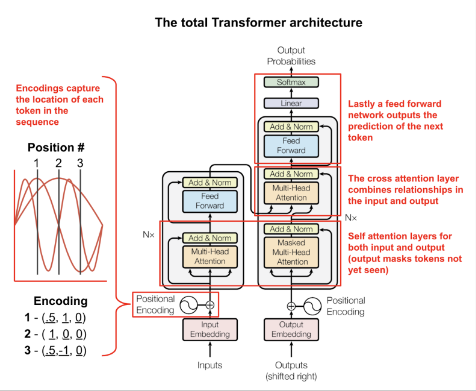
● Contextual Understanding: ChatGPT excels in maintaining context over extended conversations, something traditional models often struggle with. This is due to its ability to process large amounts of text and generate contextually relevant responses.
● Generative Capabilities: Unlike traditional models that might only classify or extract information, ChatGPT can generate creative and diverse responses, making it suitable for applications like content creation, customer service, and more.
● Adaptability: ChatGPT can be fine-tuned for specific tasks or industries, enhancing its performance across different domains.
Traditional NLP Models

Traditional NLP models include rule-based systems, statistical models, and earlier machine-learning approaches. These models often have limitations compared to newer technologies like ChatGPT.
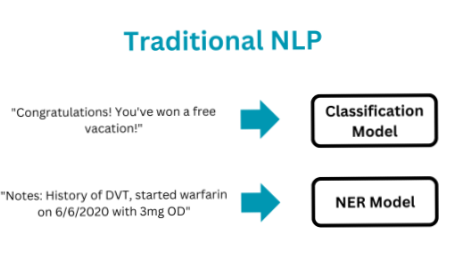
● Rule-Based Models: These use predefined rules to interpret and respond to text. While they can be effective for specific tasks, they lack the flexibility and adaptability of modern models.
● Statistical Models: These models, such as Hidden Markov Models (HMM) and Conditional Random Fields (CRF), rely on statistical methods to process language. They are limited by their reliance on manually engineered features and may not handle complex contexts well.
● Earlier Machine Learning Models: Predecessors to the Transformer-based models, like support vector machines (SVM) or logistic regression, offer limited performance in understanding and generating human-like text.
Comparative Analysis
Feature | ChatGPT | Traditional NLP Models |
Architecture | Transformer-based | Rule-based, Statistical, or earlier ML models |
Contextual Understanding | Advanced, maintains context over longer texts | Limited, often struggles with extended context |
Generative Capabilities | High, can generate diverse and coherent text | Limited, mainly focused on classification or extraction |
Flexibility | High, adaptable to various tasks and domains | Limited, often requires manual adjustments |
Training Data | Large-scale, diverse datasets | Smaller, task-specific datasets |
Training and Certification
ChatGPT Course: This course provides in-depth knowledge of the architecture, capabilities, and applications of ChatGPT. This course covers how to implement and fine-tune ChatGPT for various tasks, offering practical experience with the model.
Prompt Engineer Certification: This certification focuses on designing and optimizing prompts to interact effectively with models like ChatGPT. It equips professionals with skills to enhance the performance of conversational agents and other AI-driven applications.
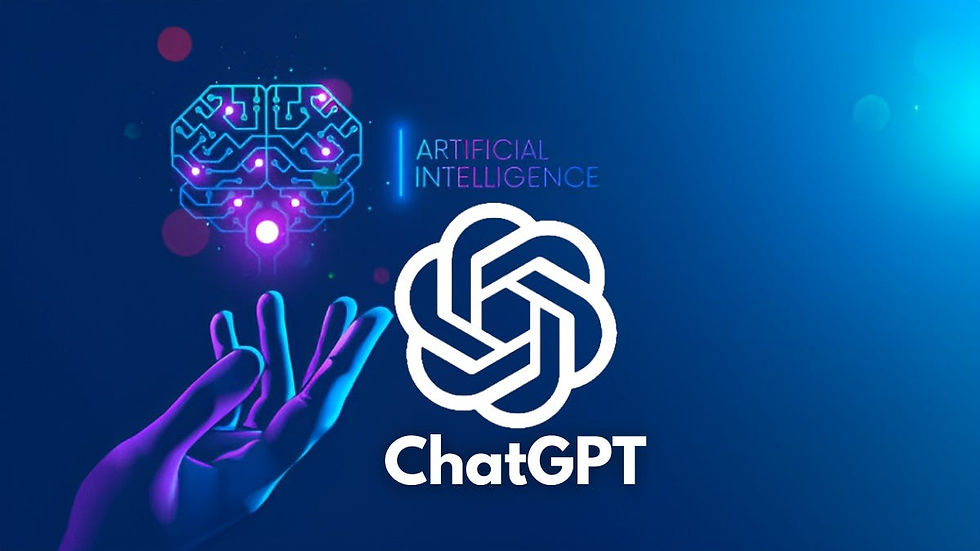
For those looking to deepen their expertise in this area, a Prompt Engineer Certification can be a valuable asset. This certification provides advanced skills in crafting and optimizing prompts, enhancing your ability to improve AI accuracy and performance across various applications.
Conclusion
The evolution from traditional NLP models to advanced systems like ChatGPT marks a significant milestone in language processing technology. While traditional models laid the groundwork, ChatGPT represents a leap forward in terms of contextual understanding, generative capabilities, and flexibility. For those interested in mastering these technologies, a ChatGPT and Prompt Engineer provide valuable expertise to leverage these advancements effectively.
Comments